Last Updated on July 18, 2024 12:03 pm by Laszlo Szabo / NowadAIs | Published on July 18, 2024 by Laszlo Szabo / NowadAIs
Patronus AI Lynx: AI’s Answer to AI Hallucinations – Key Notes
- Patronus AI launches Lynx, a leading hallucination detection model.
- Lynx outperforms GPT-4 and other models in detecting hallucinations.
- Open-source availability on Hugging Face for wider access.
- Lynx shows significant accuracy in real-world domains like medicine and finance.
- Uses advanced training techniques and datasets like HaluBench and PubMedQA.
- Partnerships with Nvidia, MongoDB, and Nomic AI for seamless integration.
Patronus AI Correct AI with AI
In the rapidly evolving landscape of large language models (LLMs), a critical challenge has emerged – the issue of hallucinations. These are instances where LLMs generate coherent but factually inaccurate responses, undermining the reliability and real-world applicability of these powerful AI systems. Recognizing the urgent need to address this problem, Patronus AI has stepped forward with a game-changing solution: Lynx, a state-of-the-art hallucination detection model that is poised to change the way enterprises leverage LLMs.
Understanding Hallucinations in LLMs
Hallucinations in LLMs occur when the models produce responses that do not align with factual reality or the provided context. This risks in domains such as medical diagnosis, financial advice, and other high-stakes applications where incorrect information can have severe consequences. Traditional LLM-as-a-judge approaches, while gaining popularity, have struggled to accurately evaluate responses to complex reasoning tasks, raising concerns about their reliability, transparency, and cost.
Introducing Lynx: The Hallucination Detection Powerhouse
1/ Introducing Lynx – the leading hallucination detection model 🚀👀
– Beats GPT-4o on hallucination tasks
– Open source, open weights, open data
– Excels in real-world domains like medicine and finance
We are excited to launch Lynx with Day 1 integration partners: @nvidia,… pic.twitter.com/FxMotNiKRQ— PatronusAI (@PatronusAI) July 11, 2024
Patronus AI’s response to this challenge is the Lynx model, a state-of-the-art hallucination detection system that outperforms even the formidable GPT-4 in a wide range of scenarios. Lynx is the first open-source model that can beat the industry-leading GPT-4 in hallucination detection tasks, showcasing its exceptional capabilities.
Lynx’s Key Features and Advantages
- Unparalleled Performance: Lynx (70B) achieved the highest accuracy in detecting hallucinations, outperforming not only OpenAI’s GPT models but also Anthropic’s Claude 3 models, all at a fraction of the size.
- Domain-Specific Expertise: Unlike previous models, Lynx and the accompanying HaluBench benchmark support real-world domains such as Finance and Medicine, making it more applicable to the challenges enterprises face.
- Explainable Reasoning: Lynx is not just a scoring model; it can also provide reasoning for its decisions, making its outputs more interpretable and transparent.
- Open-Source Accessibility: Patronus AI has made Lynx and the HaluBench dataset publicly available on Hugging Face, the open-source AI platform, democratizing access to this powerful technology.
Lynx’s Impressive Performance Across Benchmarks
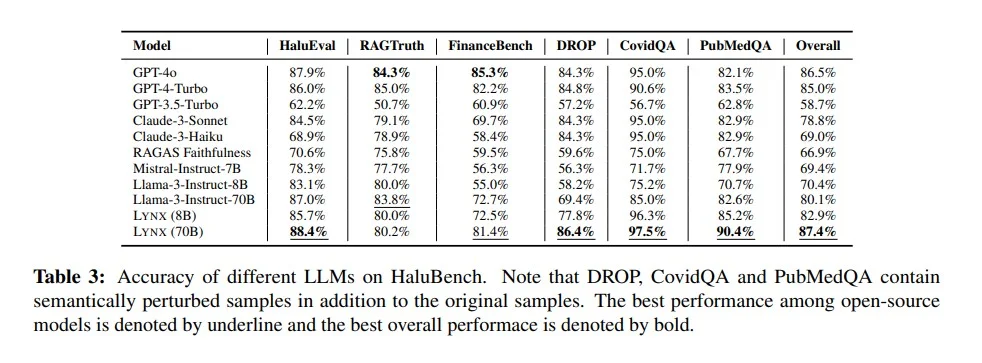
Patronus AI’s extensive testing has demonstrated Lynx’s remarkable capabilities in detecting hallucinations across various domains and scenarios.
Medical Accuracy
In the PubMedQA dataset, which evaluates medical question-answering, Lynx (70B) outperformed GPT-4o by a significant 8.3% in accurately identifying medical inaccuracies.
Hallucination Benchmark Dominance
On the comprehensive HaluBench dataset, which covers a diverse range of real-world topics, Lynx (8B) outperformed GPT-3.5 by an impressive 24.5% and surpassed the performance of Claude-3-Sonnet and Claude-3-Haiku by 8.6% and 18.4%, respectively.
Finetuning Prowess
Both the 8B and 70B versions of Lynx demonstrated significantly increased accuracy compared to open-source model baselines, with the 8B model showing a 13.3% gain over the Llama-3-8B-Instruct model through supervised finetuning.
Across-the-Board Superiority
When evaluated against GPT-3.5 across all tasks, the larger Lynx (70B) model outperformed it by an average of 29.0%, cementing its position as the most powerful open-source hallucination detection model available.
Lynx’s Innovative Training Approach
Patronus AI’s success with Lynx can be attributed to its innovative training approach, which leverages the latest advancements in language model development.
Leveraging Databricks Mosaic AI
Patronus AI utilized the Databricks Mosaic AI platform, including the LLM Foundry, Composer, and training cluster, to construct the Lynx model. This provided greater customization options and support for a wide range of language models.
Finetuning and Optimization Techniques
The Lynx-70B-Instruct model was created by finetuning the Llama-3-70B-Instruct model, with the team employing techniques such as FSDP and flash attention to enhance performance.
Comprehensive Hallucination Dataset
To create the training and evaluation datasets, Patronus AI employed a perturbation process to construct the HaluBench, a 15,000-sample benchmark that covers a diverse range of real-world topics, including Finance and Medicine.
Empowering Enterprises with Lynx
Patronus AI is committed to making Lynx and the HaluBench dataset widely accessible to enterprises, researchers, and developers, recognizing the transformative potential of this technology.
Integration Partnerships
Patronus AI has already established Day 1 integration partnerships with industry leaders like Nvidia, MongoDB, and Nomic AI, ensuring seamless integration of Lynx into a wide range of applications.
Visualization and Exploration
To further facilitate the understanding and utilization of HaluBench, Patronus AI has made the dataset available on Nomic Atlas, a powerful visualization tool that allows users to explore patterns and insights within the dataset.
The Road Ahead: Advancing Hallucination Detection
Patronus AI’s release of Lynx and HaluBench represents a great step forward in the quest to address the hallucination challenge in LLMs. By empowering enterprises with this AI technology and comprehensive evaluation platform, the company is paving the way for a new era of trustworthy and reliable AI-powered applications.
Conclusion
In a world where the proliferation of LLMs has revolutionized text generation and knowledge-intensive tasks, the issue of hallucinations has emerged as a critical obstacle. Patronus AI’s Lynx model stands as a great solution.
By open-sourcing Lynx and HaluBench, Patronus AI is driving the advancement of this crucial technology, ultimately transforming the way we interact with and trust artificial intelligence.
Definitions
- LLM Hallucinating: When large language models (LLMs) generate responses that are coherent but factually incorrect, undermining their reliability.
- HaluBench: A benchmark dataset used to evaluate AI models’ accuracy in detecting hallucinations, covering diverse real-world topics.
- PubMedQA Dataset: A dataset designed to evaluate AI models’ accuracy in medical question-answering, ensuring reliable outputs in medical contexts.
- FSDP Machine Learning Technique: Fully Sharded Data Parallelism, a technique to improve the efficiency and scalability of training large language models by distributing data and computations across multiple GPUs.
Frequently Asked Questions
1. What is Patronus AI Lynx? Patronus AI Lynx is a state-of-the-art hallucination detection model designed to identify and reduce factually incorrect responses generated by large language models, ensuring more reliable AI outputs.
2. How does Patronus AI Lynx address the issue of AI hallucinations? Lynx uses advanced training techniques and comprehensive datasets, such as HaluBench and PubMedQA, to detect hallucinations with high accuracy. This allows it to outperform models like GPT-4 in real-world scenarios.
3. What makes Lynx different from other hallucination detection models? Lynx not only excels in detecting hallucinations but also provides reasoning for its decisions, making its outputs more transparent. Additionally, it is open-source, allowing wider access and integration into various applications.
4. How can enterprises benefit from using Patronus AI Lynx? Enterprises can use Lynx to ensure their AI systems produce accurate and reliable information, particularly in high-stakes fields like finance and medicine. Lynx’s advanced capabilities and open-source availability make it a valuable tool for improving AI trustworthiness.
5. What are the key features of the HaluBench dataset used by Lynx? HaluBench is a comprehensive benchmark dataset that includes 15,000 samples covering diverse real-world topics, including finance and medicine. It is used to train and evaluate AI models’ ability to detect hallucinations accurately.