Last Updated on August 28, 2024 7:01 pm by Laszlo Szabo / NowadAIs | Published on August 28, 2024 by Laszlo Szabo / NowadAIs
Meta AI Introduces Sapiens: A New Model for Analyzing Human Actions – Key Notes
- Meta AI’s Sapiens model focuses on analyzing human actions in images and videos, excelling in complex environments.
- Sapiens handles key tasks like 2D pose estimation, body part segmentation, depth estimation, and surface normal prediction.
- Trained on over 300 million images, Sapiens performs well with limited labeled data and is expandable for enhanced performance.
- The model uses the Vision Transformers architecture and is optimized for high-resolution tasks with a variety of deployment options.
Meta AI’s Sapiens: A New AI Solution
Meta AI has introduced their new model, Sapiens, which is specifically designed to analyze and comprehend people’s actions in images and videos. This model, developed by Meta Reality Labs, offers high-resolution capabilities for human vision tasks, with a focus on understanding and analyzing people in pictures and videos. These tasks include recognizing human poses, segmenting body parts, measuring depth, and determining the orientation of objects’ surfaces. The model has been trained on over 300 million human images and can perform well in complex environments. It has also been proven to work effectively with minimal labeled data and even synthetic data, making it a valuable tool for real-world applications where data is limited. Additionally, the Sapiens model is easily expandable and performs better when the number of parameters is increased.
Key Human Vision Tasks Handled by the Sapiens Model
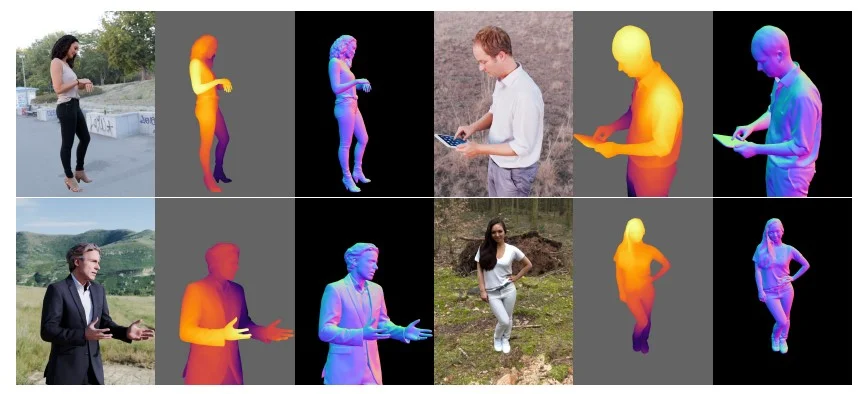
The Sapiens model is adept at handling various human vision tasks, making it versatile for different applications. It excels in tasks such as pose estimation, body part segmentation, depth estimation, and surface normal prediction, providing precise and comprehensive analysis in complex scenarios.
2D Pose Estimation: Detecting Human Movements with Precision
One of the key human vision tasks that the Sapiens model is used for is 2D pose estimation. This AI technology is essential in various fields such as video surveillance, virtual reality, motion capture, medical rehabilitation, and more, as it can recognize human poses, movements, and gestures. The model accurately detects and predicts key points of the human body, even in scenes with multiple people, making it useful for motion analysis and human-computer interaction.
Body Part Segmentation: Applications in Medical Imaging and Virtual Fitting
Another important application of the Sapiens model is body part segmentation, which is crucial in fields like medical image analysis, virtual fitting, animation production, and augmented reality. The model can accurately classify each pixel in an image into different body parts, such as the upper and lower body, facial details, etc. This feature is beneficial for developing advanced virtual clothing fitting, medical diagnostic tools, and more natural virtual character animation.
Depth Estimation: Understanding 3D Structures in Complex Scenes
Depth estimation is another key task where the Sapiens model excels. This is essential in autonomous driving, robot navigation, 3D modeling, and virtual reality as it helps understand the scene’s three-dimensional structure. The model is capable of inferring depth information from a single image, especially in human scenes. This feature supports various applications that require an understanding of spatial relationships, such as obstacle detection in autonomous driving and robot path planning.
Surface Normal Prediction for High-Quality 3D Rendering
Surface normal prediction, which is widely used in 3D rendering, physical simulation, reverse engineering, and lighting processing, is also a specialty of the Sapiens model. It can infer the surface normal direction of each pixel in an image, which is crucial for generating high-quality 3D models and achieving more realistic lighting effects. This function is particularly important in applications that require precise surface features, such as virtual reality and digital content creation.
Versatility of Sapiens in Diverse Human-Centric Vision Tasks
The Sapiens model’s versatility is evident in its ability to handle various human-centric vision tasks, making it a useful tool in scenarios like social media content analysis, security monitoring, sports science research, and digital human generation. Due to its strong performance on multiple tasks, the Sapiens model can serve as a general base model to support different human-centric vision tasks, accelerating the development of related applications.
Enhancing Virtual and Augmented Reality Experiences with Sapiens
Virtual reality and augmented reality applications require an accurate understanding of human posture and structure to create an immersive experience. The Sapiens model provides high-resolution, accurate human poses and part segmentation, making it suitable for creating realistic human images in virtual environments. It can also dynamically adapt to changes in user movements.
Sapiens in Medical and Health Applications: Improving Patient Care
In the medical and health field, the Sapiens model’s accurate posture detection and human segmentation can aid in patient monitoring, treatment tracking, and rehabilitation guidance. This model helps medical professionals analyze patients’ posture and movement to provide personalized and effective treatment plans.
Technical Features and Methods Used in the Sapiens Model
The Sapiens model’s technical methods include the use of the Humans-300M dataset for pre-training, which contains 300 million “in-the-wild” human images. The dataset has been carefully curated to ensure data quality. The model also uses the Vision Transformers (ViT) architecture, which divides the image into non-overlapping patches for better handling of high-resolution inputs. The model’s architecture consists of an encoder for feature extraction and a decoder for task-specific functions. Pre-training is done using the Masked Autoencoder (MAE) method, which enables the model to learn more robust feature representations. The model is also pre-trained on high-quality labeled data and uses multiple tasks such as 2D pose estimation, body part segmentation, depth estimation, and surface normal prediction. It is optimized using the AdamW optimizer with cosine annealing and linear decay learning rate strategies. Finally, the model demonstrates extensive zero-shot generalization capabilities, even with limited training data.
Conclusion: Sapiens as a Valuable Tool for Human-Centric Vision Tasks
In conclusion, the Sapiens model is a powerful tool for various human-centric vision tasks, with its ability to accurately analyze and understand people’s actions in images and videos. Its performance has been proven to surpass existing methods, making it a valuable asset in different applications.
Descriptions
- 2D Pose Estimation: A process where the model detects and predicts key points of the human body, such as joints, to determine poses and movements. It’s crucial for fields like virtual reality and medical rehabilitation.
- Body Part Segmentation: The classification of each pixel in an image into different body parts, helping in applications like medical imaging, virtual fittings, and augmented reality to achieve detailed understanding and visualization.
- Depth Estimation: A technique that infers the distance of objects in an image, helping machines understand three-dimensional spaces. Useful in robotics, autonomous driving, and virtual reality for tasks like obstacle detection.
- Surface Normal Prediction: The determination of the direction perpendicular to a surface at each pixel, aiding in 3D rendering and achieving realistic lighting effects in virtual simulations and digital creations.
- Vision Transformers (ViT): An AI architecture that processes images by dividing them into smaller patches, allowing for better handling of high-resolution inputs and improving model accuracy and efficiency.
- Masked Autoencoder (MAE): A training technique where parts of the input are masked or hidden, enabling the model to learn more robust representations by predicting the missing parts.
- Zero-Shot Generalization: The model’s ability to apply learned knowledge to new, unseen tasks without additional training, demonstrating versatility and adaptability.
Frequently Asked Questions
- What is Meta AI’s Sapiens model? Meta AI’s Sapiens is a model designed to analyze human actions in images and videos. It excels in tasks like pose estimation, body part segmentation, and depth estimation to understand complex visual data.
- How does Meta AI’s Sapiens perform 2D pose estimation? The Sapiens model uses advanced algorithms to detect and predict key points of the human body, identifying poses and movements even in crowded scenes. This makes it ideal for surveillance, virtual reality, and healthcare.
- Why is body part segmentation important in Meta AI’s Sapiens? Body part segmentation helps categorize each pixel into specific body parts, which is crucial for applications like medical imaging, virtual clothing fitting, and creating lifelike animations in augmented reality.
- How does depth estimation work in Meta AI’s Sapiens? Depth estimation in Sapiens allows the model to understand the three-dimensional structure of a scene from a single image. It is useful for robot navigation, autonomous driving, and creating immersive virtual environments.
- What technologies power the Sapiens model? Meta AI’s Sapiens is built on the Vision Transformers (ViT) architecture and uses a Masked Autoencoder (MAE) for training. It leverages a large dataset and sophisticated algorithms for robust performance.