Last Updated on May 7, 2024 12:28 pm by Laszlo Szabo / NowadAIs | Published on May 7, 2024 by Laszlo Szabo / NowadAIs
Google’s Med-Gemini AI is Better at Diagnosing Than Doctors – Key Notes
- Advanced AI Integration: Med-Gemini integrates advanced AI to tackle complex medical data across images, videos, and EHRs.
- Specialized Components: Incorporates custom encoders for processing health signals like ECGs and utilizes chain-of-reasoning methodologies.
- Benchmark Achievements: Achieved 91.1% accuracy on the MedQA (USMLE) benchmark, outperforming existing models like Med-PaLM 2.
- Multimodal Mastery: Excelled in handling complex medical information across multiple modalities, providing comprehensive medical insights.
- Clinical Reasoning and Safety: Enhances clinical decision-making with uncertainty-guided web search and ensures safety with specialized encoders.
Google’s Med-Gemini is Here
The integration of artificial intelligence (AI) into the healthcare domain is fundamentally transforming the landscape of medical practices, from diagnosis to treatment planning and research assimilation. Advanced AI models hold immense potential to elevate healthcare standards by bolstering accuracy and efficiency. However, the complexity of medical data, spanning diverse modalities such as images, videos, and electronic health records (EHRs), poses a formidable challenge for AI systems, necessitating adept comprehension and interpretation capabilities.
Existing AI models, including large language models (LLMs), often struggle to seamlessly analyze medical data, grappling with challenges in understanding multimodal information, synthesizing extensive contextual records, and accurately retrieving medical insights from diverse sources. As a result, there is a pressing need for specialized AI tools designed to proficiently handle medical data while offering precise real-time support to medical practitioners.
The Med-Gemini Breakthrough
Addressing these exigencies, the collaborative efforts of Google Research, Google DeepMind, Google Cloud, and Verily have yielded Med-Gemini family of models. These models, an extension of the Gemini 1.0 and 1.5 architectures, incorporate specialized components tailored for medical applications, setting a new benchmark in the realm of medical AI.
Enhancing Clinical Reasoning
Med-Gemini’s advancements build upon the foundation of the Gemini architecture, introducing innovative features that enhance clinical decision-making. One such feature is the incorporation of uncertainty-guided web search, which facilitates precise medical query resolution by seamlessly integrating online information with the model’s internal knowledge.
Additionally, Med-Gemini boasts custom encoders adept at processing health-related signals, such as electrocardiograms (ECGs), further augmenting its capabilities. Moreover, the integration of chain-of-reasoning methodologies empowers the models to process and decipher extensive medical records, ensuring nuanced understanding and accurate responses.
Surpassing Benchmarks
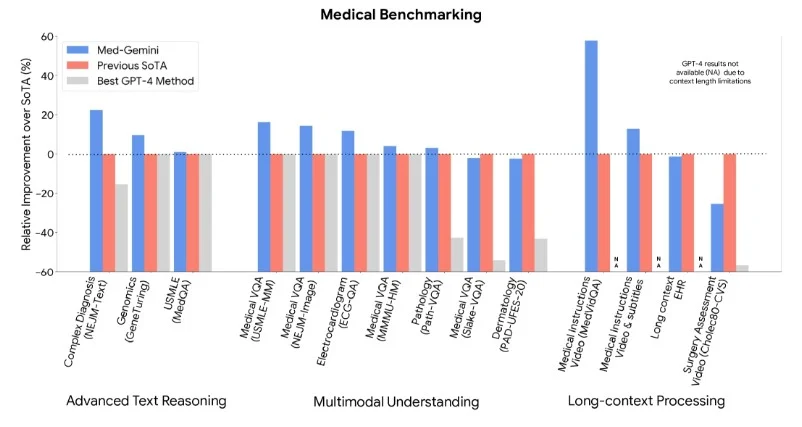
Demonstrating substantial progress, Med-Gemini models have eclipsed prior benchmarks, achieving state-of-the-art results across 14 benchmarks encompassing 25 tasks. Notably, they have outperformed counterparts like GPT-4 and Med-PaLM 2, boasting a remarkable 91.1% accuracy on the MedQA (USMLE) benchmark, surpassing Med-PaLM 2 by 4.6%.
Multimodal Mastery
The exceptional performance of Med-Gemini extends beyond textual understanding, as the models exhibit greatt enhancements in multimodal tasks. They have demonstrated large improvements in medical image and video analysis, alongside precise information retrieval from extensive health records, underscoring their prowess in accurate medical reasoning.
Addressing Limitations of Existing AI Models
The challenges faced by existing AI models in the healthcare domain are multifaceted. LLMs, for instance, often struggle with medical inquiries and processing diverse data types like medical images and videos. Moreover, their effectiveness in synthesizing information from lengthy EHRs remains suboptimal, highlighting the need for specialized AI tools.
Overcoming Multimodal Challenges
One of the key limitations of current AI models is their difficulty in comprehending multimodal information, which is prevalent in medical data. Med-Gemini addresses this challenge by incorporating custom encoders and chain-of-reasoning techniques that enable seamless integration and interpretation of diverse data modalities, including images, videos, and structured health records.
Enhancing Long-Context Processing
Another crucial limitation of existing AI models is their suboptimal performance in processing and synthesizing information from extensive medical records. Med-Gemini’s advancements in long-context processing, facilitated by its chain-of-reasoning methodologies, empower the models to navigate and decipher these voluminous data sources, ensuring nuanced understanding and accurate responses.
Improving Clinical Reasoning
Existing AI models, including LLMs, often struggle with accurate clinical reasoning, particularly in complex medical scenarios. Med-Gemini’s incorporation of uncertainty-guided web search and specialized encoders for health-related signals equips the models with enhanced clinical decision-making capabilities, enabling them to provide more reliable and informed recommendations.
Revolutionizing Healthcare Delivery
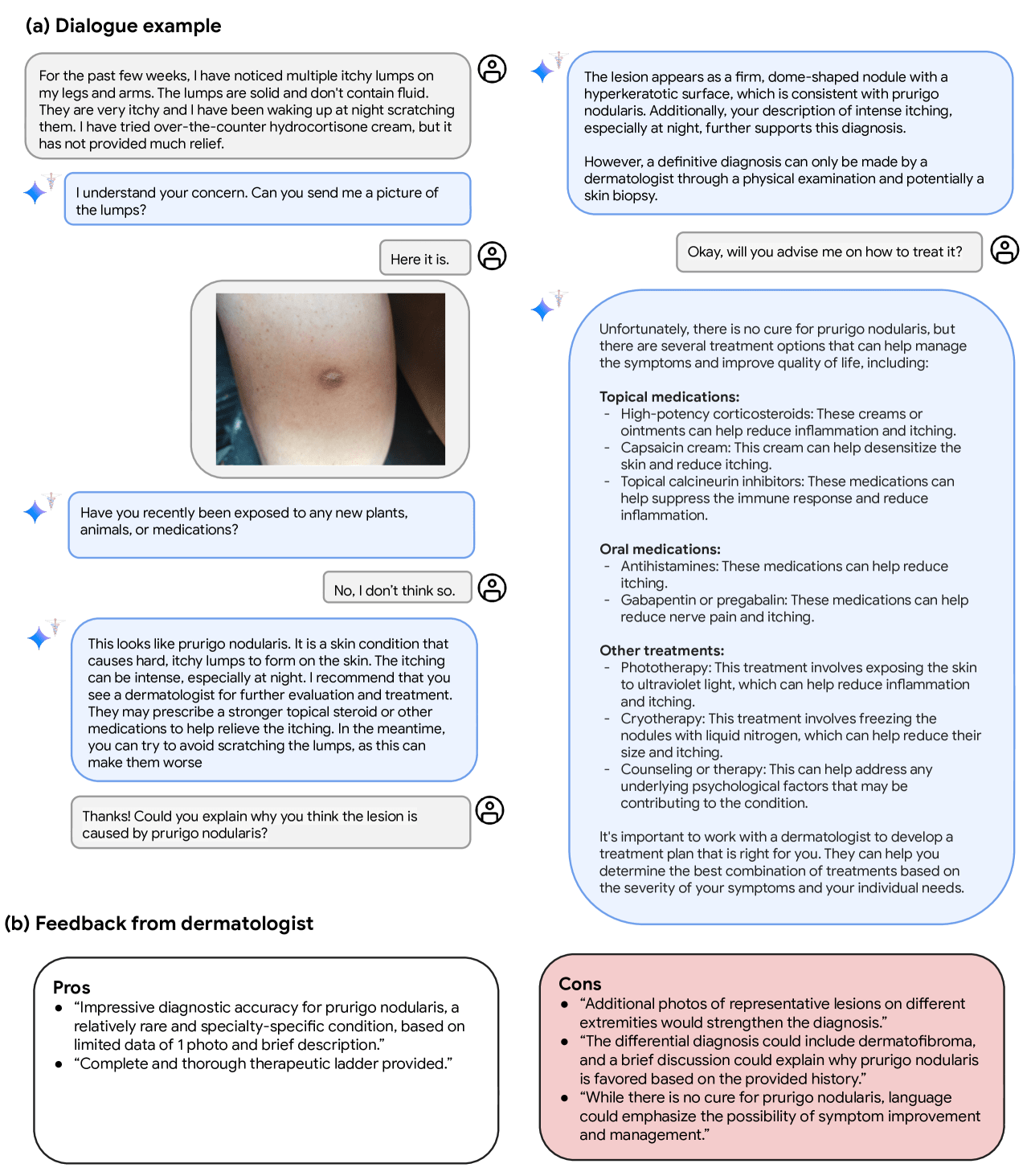
The introduction of the Med-Gemini family of models by Google DeepMind leads advancement in medical AI. With its enhanced capabilities in clinical reasoning, multimodal understanding, and long-context processing, Med-Gemini sets a new standard for AI models in healthcare.
Enhancing Diagnostic Accuracy
Med-Gemini’s exceptional performance in medical image and video analysis, coupled with its precise information retrieval from health records, holds immense potential to revolutionize the diagnostic process. By providing more accurate and comprehensive insights, these models can assist medical professionals in making more informed decisions, leading to improved patient outcomes.
Streamlining Treatment Planning
The Med-Gemini models’ ability to synthesize and interpret extensive medical data can also greatly benefit the treatment planning process. By offering more nuanced and context-aware recommendations, these AI tools can help healthcare providers develop personalized treatment plans that cater to the unique needs of each patient.
Accelerating Research Assimilation
The integration of Med-Gemini’s capabilities in medical AI can also expedite the assimilation of the latest research findings into clinical practice. By efficiently processing and interpreting the vast array of medical literature, these models can empower healthcare professionals to stay abreast of the latest advancements, ultimately enhancing the quality of care delivered to patients.
Responsible AI Development
As Med-Gemini continues to push the boundaries of medical AI, Google has emphasized the importance of integrating responsible AI principles throughout the model development process. This includes addressing critical aspects such as fairness, privacy, equity, transparency, and accountability.
Ensuring Ethical Deployment
Google researchers have acknowledged the need for further fine-tuning and specialization of the Med-Gemini models before they can be safely deployed in real-world healthcare settings. The commitment to responsible AI development underscores the organization’s dedication to ensuring the ethical and transparent use of these powerful technologies.
Fostering Collaborative Innovation
The collaborative nature of the Med-Gemini project, involving researchers from Google Research, Google DeepMind, Google Cloud, and Verily, highlights the importance of interdisciplinary cooperation in driving innovation within the medical AI landscape. By leveraging diverse expertise and perspectives, these teams are poised to continue pushing the boundaries of what is possible in the realm of healthcare technology.
Conclusion
The introduction of the Med-Gemini family of models by Google DeepMind represents a significant milestone in the evolution of medical AI. With its enhanced capabilities in clinical reasoning, multimodal understanding, and long-context processing, Med-Gemini sets a new benchmark in healthcare technology, promising to revolutionize various aspects of medical practice, from diagnosis to treatment planning and research assimilation.
As the healthcare industry continues to embrace the transformative power of AI, the Med-Gemini models serve as a testament to the potential of specialized, tailored AI solutions to elevate the standards of care and improve patient outcomes. With a steadfast commitment to responsible development and collaborative innovation, the future of medical AI, as exemplified by Med-Gemini, holds immense promise for a healthier and more equitable healthcare landscape.
Definitions
- Med-Gemini: A family of models developed by Google that leverages advanced AI to improve clinical decision-making and medical data analysis.
- Electronic Health Records (EHRs): Digital versions of patients’ medical histories maintained over time, including diagnoses, medications, treatment plans, immunization dates, allergies, and test results.
- Electrocardiograms (ECGs): Tests that record the electrical activity of the heart over a period to help diagnose various heart conditions.
- MedQA (USMLE) Benchmark: A medical question-answering benchmark used to evaluate AI systems on their ability to understand and answer questions typically found in the United States Medical Licensing Examination (USMLE).
- Med-PaLM 2: An advanced AI model specifically developed for medical applications, previously one of the top-performing models in medical benchmarks before Med-Gemini.
Frequently Asked Questions
- What is Google’s Med-Gemini designed to achieve in healthcare? Google’s Med-Gemini aims to enhance medical diagnostic accuracy and streamline treatment planning through AI. By processing complex medical data efficiently, it supports healthcare providers in delivering personalized, accurate care.
- How does Google’s Med-Gemini handle complex medical data like EHRs and ECGs? Med-Gemini utilizes specialized components to interpret diverse data types such as EHRs and ECGs. This allows the model to provide comprehensive analyses that are crucial for accurate diagnostics and effective treatment planning.
- What makes Google’s Med-Gemini different from other medical AI models? Unlike other models, Med-Gemini integrates multimodal data and achieves high accuracy across numerous medical benchmarks. Its ability to synthesize extensive medical information and provide actionable insights sets it apart.
- Can Google’s Med-Gemini improve clinical decision-making? Yes, Med-Gemini enhances clinical decision-making by incorporating an uncertainty-guided web search that helps validate and refine the AI’s recommendations, ensuring more reliable and precise medical advice.
- What are the future directions for Google’s Med-Gemini in healthcare? Future enhancements for Med-Gemini include further integration of multimodal data and improving real-time response capabilities. Ongoing developments aim to refine its diagnostic precision and expand its application across different areas of healthcare.