Last Updated on December 19, 2023 10:50 am by Laszlo Szabo / NowadAIs | Published on December 19, 2023 by Laszlo Szabo / NowadAIs
Generative AI in Drug Discovery: Transforming Medical Research – Key Notes Section:
- Generative AI greatly speeds up the drug discovery process through advanced data analysis and prediction models.
- It plays a key role in molecular design, target identification, and drug repurposing.
- AI technologies like deep learning are central to Generative AI’s effectiveness.
- Generative AI contributes to significant time and cost savings in drug development.
- Personalized medicine is greatly enhanced by AI’s ability to tailor treatments to individual genetic profiles.
- Implementation challenges include data accuracy, computational demands, and ethical considerations.
- Patient safety is bolstered through AI’s accurate predictions of drug interactions and side effects.
- The future of Generative AI in drug discovery looks promising, with continual advancements in AI technologies.
Introduction to Generative AI in Drug Discovery
The incorporation of Generative AI in Drug Discovery marks a significant shift in pharmaceutical research. This technological advancement is redefining the landscape of drug development, offering unprecedented efficiencies and possibilities.
The Advent of AI in Pharmaceutical Research
The journey of AI in pharmaceuticals began as a support tool but has rapidly evolved into a key player. Today, AI-Driven Pharmaceutical Research is not just supplementing human efforts; it’s reshaping the entire process. From Molecular Design with AI to AI for Drug Target Identification, these technologies are enabling faster and more precise drug development.
The Scope and Potential of Generative AI
Generative AI’s potential in drug discovery is immense. By automating and optimizing processes such as AI Predictions in Drug Interactions and Drug Repurposing Using AI, it’s leading to more efficient research methodologies. The technology’s ability to analyze vast datasets quickly helps identify potential drug candidates faster than traditional methods. Furthermore, its role in Personalized Medicine and AI is groundbreaking, tailoring treatments to individual genetic profiles.
Generative AI also emphasizes the importance of ethics in its application. AI in Drug Development Ethics is a critical conversation, ensuring that the development of AI for Novel Medicines is conducted responsibly. The technology’s contribution to AI and Genetic-Based Treatments is a testament to its potential to enhance healthcare outcomes significantly.
In summary, Generative AI in Drug Discovery is not just an innovation; it’s a paradigm shift. By improving efficiency, accuracy, and personalization, AI is not only speeding up the drug discovery process but also enhancing patient safety. The future of pharmaceutical research, driven by AI, holds promising prospects for the development of new, effective treatments, marking a new era in healthcare.
Accelerating Molecular Design through Generative AI
The application of Generative AI in Drug Discovery has profoundly impacted the field of pharmaceutical research. By leveraging advanced AI-driven techniques, scientists are now able to propose novel molecular structures for potential drugs more rapidly than ever before. This leap in technology facilitates the exploration of new territories in molecular design, often leading to the discovery of compounds with unique and efficacious properties.
One of the key aspects of this evolution is the use of AI in predicting molecular properties. AI systems, through sophisticated algorithms, can analyze extensive chemical databases, enabling them to forecast the behavior and efficacy of new compounds. This approach not only accelerates the design process but also enhances the precision in targeting specific medical conditions.
The implementation of deep learning and machine learning in drug discovery, particularly in quantitative structure-activity relationships (QSAR), has further bolstered the ability to predict drug-target interactions and molecular properties with unprecedented accuracy. These AI methodologies are not just theoretical constructs but are being actively used in the development of new therapeutic agents, as seen in recent preclinical studies targeting specific proteins involved in ovarian cancer.
Moreover, the AI-designed molecules often exhibit unique characteristics that differ significantly from traditionally designed compounds. This novelty stems from AI’s ability to traverse unexplored regions of chemical space, bringing forth innovative solutions to complex pharmacological challenges. The introduction of these ‘weird’ molecules, as some scientists refer to them, opens up new possibilities in drug design that were previously inconceivable.
Generative AI’s Impact on Drug Development
The implications of Generative AI in drug discovery are far-reaching. Not only does it expedite the molecular design process, but it also allows for a more thorough exploration of chemical space, leading to the discovery of novel drug candidates. These advancements are reshaping the landscape of pharmaceutical research, offering the promise of more effective, personalized medicines and a faster path to drug development.
In conclusion, Generative AI in Drug Discovery is not just a futuristic concept but a present reality that is dynamically transforming how new medicines are designed and developed. This AI-driven approach is setting new benchmarks in the pharmaceutical industry, heralding a new era of drug discovery that is more efficient, innovative, and promising for future medical advancements.
Accelerating Molecular Design through Generative AI
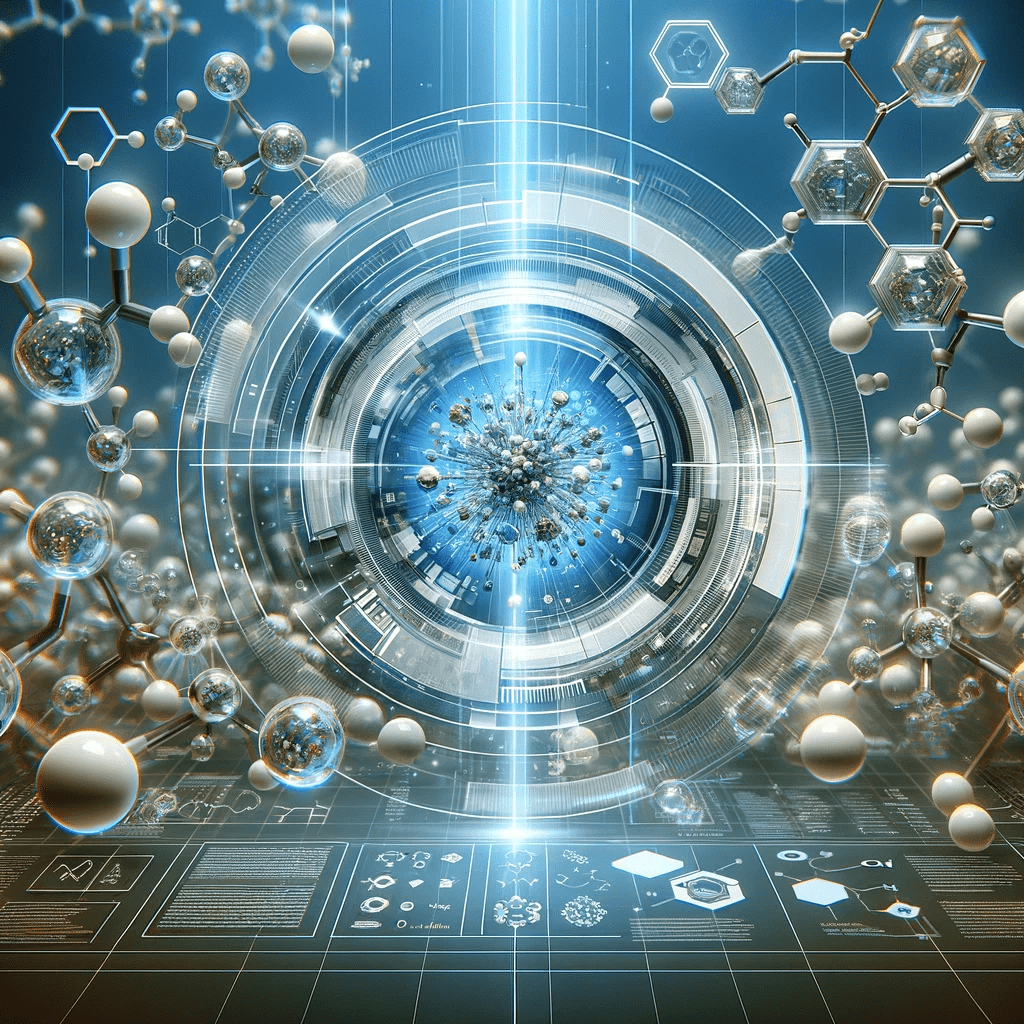
The role of generative AI in drug discovery, particularly in accelerating molecular design, is revolutionizing the pharmaceutical industry. Here’s an overview focusing on novel molecular structures and the prediction of molecular properties:
Generative AI in Drug Discovery: Unlocking New Potentials in Molecular Design
Generative AI, with its deep learning capabilities, is making significant strides in de novo molecular design. This involves the creation of new synthetic data, including molecular structures, which are pivotal in developing new drugs. Deep generative models, a subset of AI, are particularly effective in this domain, showing superior performance in generating new molecular designs.
Novel Molecular Structures for Future Medicines
One of the most remarkable applications of generative AI is in designing novel molecular structures. By synthesizing and analyzing vast amounts of data, AI algorithms can propose new molecular compounds that could lead to effective treatments for various diseases. This process not only speeds up the discovery of new drugs but also opens up possibilities for treatments that were previously unthought of.
The Role of AI in Predicting Molecular Properties
In addition to creating new molecular structures, generative AI plays a crucial role in predicting the properties of these molecules. This includes assessing their potential efficacy, safety, and interaction with other drugs. By accurately predicting these properties, AI helps in identifying promising drug candidates more efficiently and reduces the risks associated with drug development.
Generative AI’s impact on molecular design and property prediction is transforming drug discovery, making it more efficient, cost-effective, and innovative. As we continue to see advancements in this field, the potential for AI-driven pharmaceutical research to bring forth groundbreaking treatments is immense.
Generative AI in Drug Discovery: Transforming Target Identification and Validation
In the dynamic field of pharmaceutical research, Generative AI in Drug Discovery is emerging as a game-changer, particularly in target identification and validation. This technology leverages the power of artificial intelligence to revolutionize traditional approaches, offering accelerated pathways for novel treatments.
Harnessing Big Data for Disease Targeting
Generative AI models, with their capability to create new data samples based on patterns in training data, are pivotal in unveiling novel molecular structures or drug candidates. These models, which include advanced techniques like generative adversarial networks and variational autoencoders, contrast with predictive AI that relies on existing data to make predictions. In the realm of AI-Driven Pharmaceutical Research, this technology optimizes molecular structures and analyzes large datasets, leading to significant time savings in drug discovery processes. Notably, technologies like AlphaFold have demonstrated their potential by discovering novel small molecule inhibitors in a remarkably short time, significantly reducing the traditional discovery process from years to months.
Enhancing Drug Development Success Rates
The application of Generative AI in drug discovery extends to improving success rates in the drug development pipeline. For example, companies like Exscientia have demonstrated how using AI for small-molecule drug discovery can identify drug candidates in significantly less time than traditional methods. This process, which encompasses Molecular Design with AI and AI Predictions in Drug Interactions, not only accelerates drug discovery but also holds the potential for cost savings. By automating certain aspects of drug development, generative AI reduces labor-intensive tasks, curbs the need for manual labor, and minimizes human error rates.
Generative AI also fosters interdisciplinary collaboration, harmonizing data from heterogeneous sources and fueling collaborative data networks. This approach is essential for AI in Drug Development Ethics, ensuring that diverse and representative datasets are used to minimize potential biases.
However, the implementation of Generative AI in drug discovery is not without challenges. Concerns regarding data privacy and ethics, lack of standardization, and dependence on biased or incomplete data are critical. To address these, companies must establish clear policies, interact with regulatory authorities, and create ethical frameworks to guide the use of AI. Furthermore, developing standardized protocols and ensuring high-quality data curation are essential to mitigate risks related to biased predictions and AI hallucination.
Generative AI and Drug-Drug Interaction Predictions: Ensuring Safer Drug Combinations and Enhancing Patient Safety
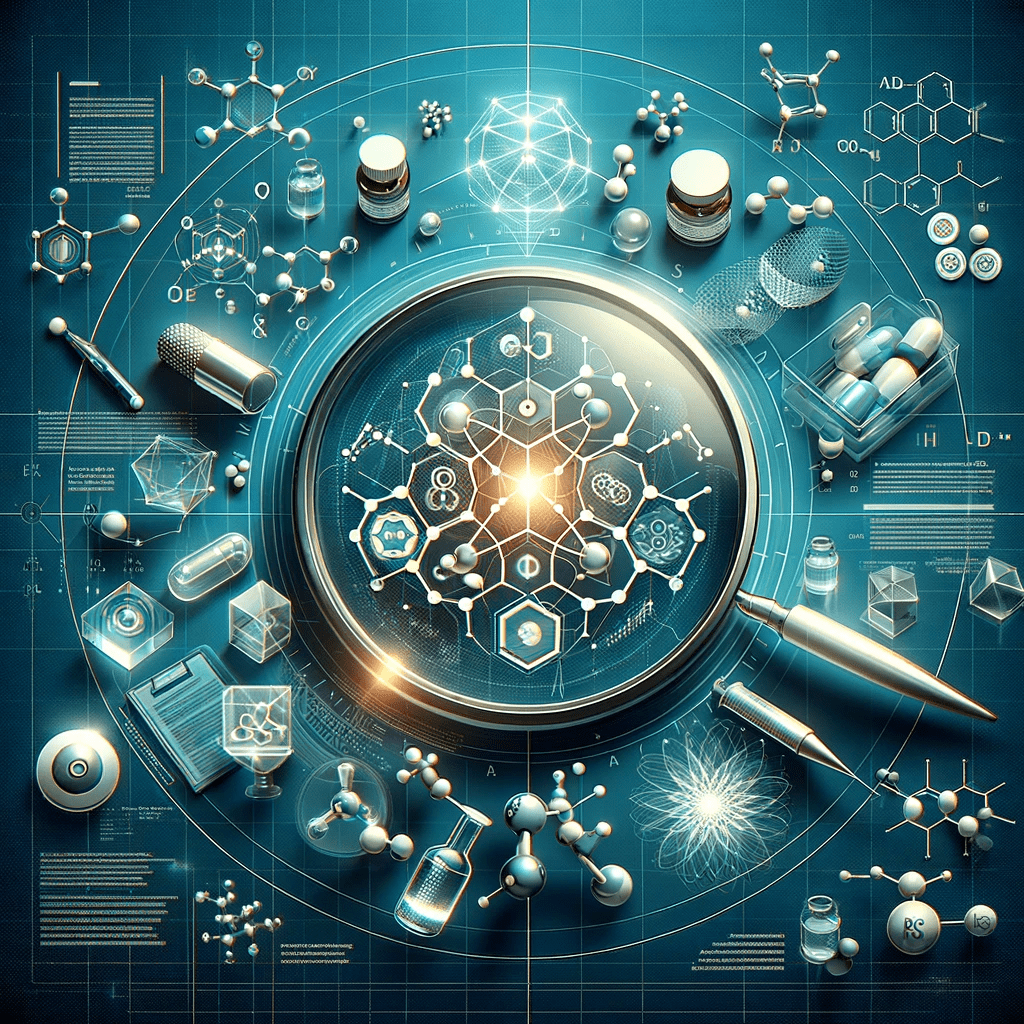
Generative AI in drug discovery is significantly transforming the pharmaceutical landscape, especially in predicting drug-drug interactions, ensuring safer drug combinations, and enhancing patient safety.
Ensuring Safer Drug Combinations
Generative AI models are proficient in predicting potential drug-drug interactions. This capability is vital for patient safety, as it helps in avoiding harmful side effects and optimizing treatment regimens. For example, the DiffDock model developed by MIT researchers employs a unique approach to computational drug design. This model identifies multiple binding sites on proteins, which is crucial in understanding how drugs will interact with each other and with the body. By predicting these interactions, Generative AI aids in creating safer and more effective combination therapies.
Impact on Treatment Regimens and Patient Safety
The adoption of Generative AI in drug discovery not only accelerates the process of identifying effective drug combinations but also plays a significant role in enhancing patient safety. The ability to predict how different drugs will interact within the body allows for the optimization of treatment regimens. This optimization is particularly important in complex cases where patients may be taking multiple medications.
Moreover, the use of Generative AI models like DiffDock opens up new opportunities in the realm of “blind docking,” which can significantly improve the process of drug target identification. This improvement is crucial for identifying potential “off-target” effects early on, thus preventing adverse effects during clinical trials. The integration of protein folding techniques with Generative AI, as seen in the use of AlphaFold 2 by DeepMind, further aids in understanding drug mechanisms of action, which is a key factor in ensuring patient safety.
In conclusion, Generative AI in drug discovery is not just an innovative technological advancement; it’s a transformative force reshaping the approach to molecular design, drug target identification, and the overall safety and effectiveness of pharmaceuticals. As Generative AI continues to evolve, it holds the potential to significantly impact patient treatment outcomes and the efficiency of the drug development process.
The Emergence of Drug Repurposing through AI
The emergence of Generative AI in Drug Discovery has opened new avenues in pharmaceutical research, particularly in the field of drug repurposing. This innovative approach leverages AI-driven technologies to identify new therapeutic uses for existing drugs, a process that is reshaping the landscape of modern medicine.
New Uses for Existing Drugs
Drug Repurposing Using AI has become a crucial strategy in modern pharmaceuticals, offering a cost-effective and efficient method to extend the lifecycle of existing drugs. By analyzing vast amounts of data, AI for Drug Target Identification and Molecular Design with AI can uncover previously unknown applications of existing medications, thereby accelerating the development of novel therapies. For instance, drugs initially developed for one disease may show potential in treating completely different conditions. This approach not only saves time and resources but also provides new hope for patients with complex diseases.
Cost-Effectiveness in Drug Development
One of the most significant advantages of Generative AI in Drug Discovery is its impact on the cost and efficiency of drug development. Traditional drug discovery processes are notoriously time-consuming and expensive. However, AI Predictions in Drug Interactions and Drug Repurposing Using AI significantly reduce these barriers, making drug development more accessible and sustainable. Moreover, this approach minimizes the risks associated with the development of entirely new drugs, as repurposed drugs have already undergone extensive testing and approval processes.
Generative AI in Drug Discovery is not without its challenges. Issues like ensuring selectivity, managing toxicity, and understanding the intricate balance between efficacy and side effects are critical considerations. Despite these challenges, AI in drug repurposing holds immense potential, particularly in the development of Personalized Medicine and AI and AI and Genetic-Based Treatments, which are increasingly important in modern healthcare.
In conclusion, Generative AI in Drug Discovery is reshaping the pharmaceutical landscape, offering innovative solutions for drug repurposing and enhancing patient safety. By capitalizing on the capabilities of AI, researchers can discover new applications for existing drugs, paving the way for more effective and personalized treatments.
Generative AI in Drug Discovery: Personalizing Treatment Through Genetic Insight
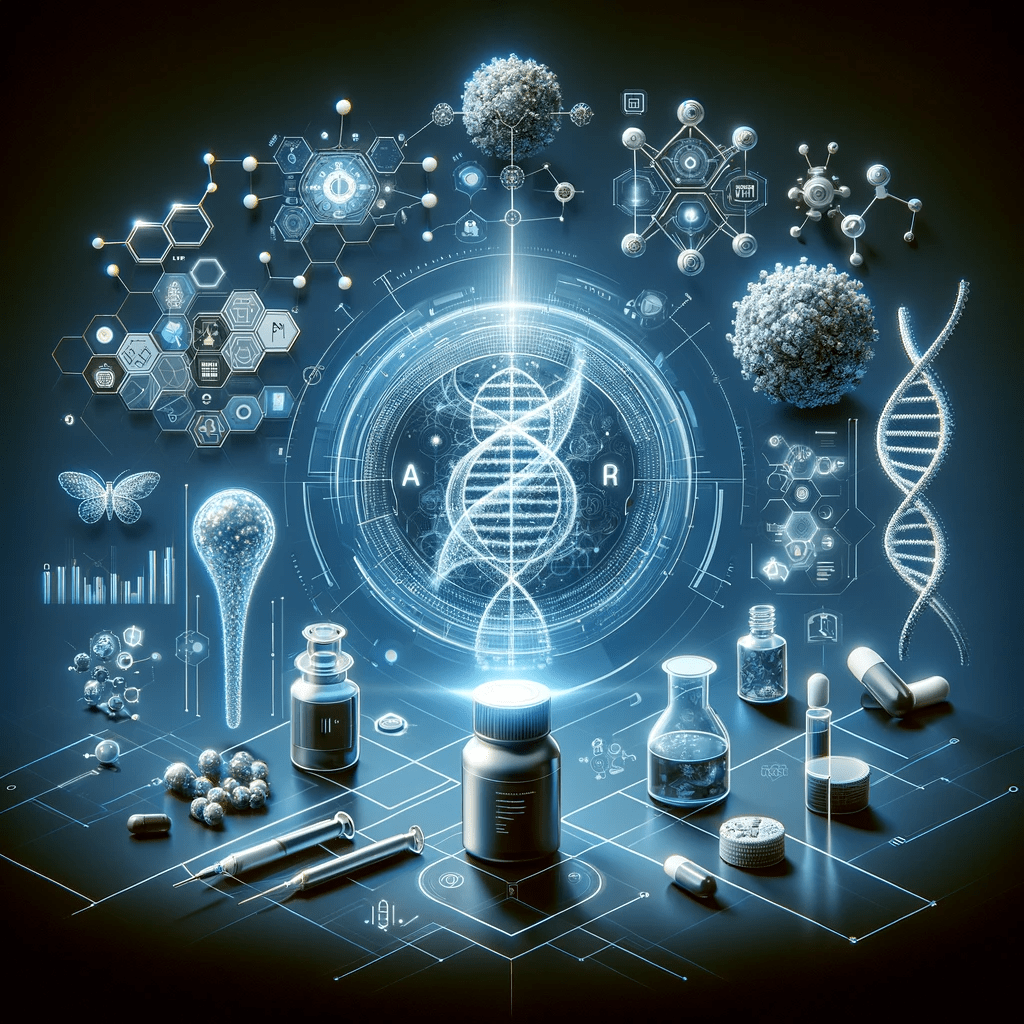
Personalized Medicine and AI: Tailoring Treatments for Individual Needs
Tailored Treatments Based on Genetic Makeup Generative AI in Drug Discovery is now at the forefront of personalized medicine, offering AI-generated therapy regimens based on an individual’s genetic and medical history. This approach in Personalized Medicine and AI harnesses the power of AI to analyze vast amounts of data, creating AI and Genetic-Based Treatments that are uniquely suited to each patient. The AI system gathers and utilizes patient-specific data, including genetic information, to offer healthcare recommendations, thereby aiding healthcare professionals in clinical decision-making.
Advancing Towards Individualized Healthcare Solutions
The incorporation of AI into personalized treatment signifies a major advancement in Molecular Design with AI and AI for Drug Target Identification. This advancement is crucial in enhancing patient safety and efficacy of treatments. By focusing on the patient’s unique genetic makeup, AI Predictions in Drug Interactions and Drug Repurposing Using AI become more precise, leading to safer and more effective treatment outcomes.
Generative AI in Drug Discovery is not just a tool for creating novel medicines but a pathway towards more personalized and patient-centric healthcare solutions. Its integration into the healthcare system requires adjustments in infrastructure but promises significant improvements in the precision and efficacy of treatments.
Navigating the Challenges and Ethical Considerations in Generative AI in Drug Discovery
Safety and Efficacy of AI-Generated Drug Candidates
The realm of Generative AI in Drug Discovery has brought about a paradigm shift in how new medicines are developed. However, ensuring the safety and efficacy of AI-generated drug candidates remains a paramount concern. Generative AI models, trained on extensive datasets, are capable of generating novel molecular structures and predicting drug interactions. But the challenge lies in validating the safety and effectiveness of these AI-generated compounds before they can be considered for clinical trials.
Addressing Data Biases and Ethical Concerns
Another critical aspect of Generative AI in Drug Discovery involves navigating the ethical landscape, particularly addressing issues like algorithmic bias and data integrity. Algorithmic bias in AI models can lead to skewed results, which is especially concerning in healthcare where it may result in patient mistreatment. Ensuring that AI systems are trained on diverse and unbiased data sets is crucial for the development of fair and effective AI-driven pharmaceutical solutions.
Generative AI in healthcare also raises questions about patient privacy and the handling of sensitive health information. The interactive nature of generative AI, such as those used in medical chatbots or diagnosis tools, increases the risk of misinformation and privacy breaches. Thus, ethical considerations around data use, patient privacy, and the reliability of AI predictions are at the forefront of discussions in AI-driven drug discovery.
The Future of Drug Discovery with Generative AI
The Transformative Impact on Medical Research
Generative AI in drug discovery has significantly transformed the medical research landscape. Its integration into the drug development process is shifting the traditional, human-centric approach towards a more consistent and precise methodology. This shift is crucial in reducing human errors and variability, which have often been stumbling blocks in traditional drug discovery. The iterative structure of AI in this context means that failures at any stage can be quickly identified and rectified, leading to a more efficient process and reduced costs.
AI’s role in bridging knowledge gaps is also noteworthy. The complex expertise required in fields like chemistry and data science can be overwhelming for individual scientists. AI tools make these complex fields more accessible, reducing barriers and enhancing efficiency. By placing AI tools directly in the hands of scientists, the entire drug discovery process becomes more streamlined, enabling scientists to rapidly test ideas and advance them through the development pipeline.
The Vision for Improved Patient Well-being and Treatment
Generative AI in drug discovery is not just about improving processes; it’s fundamentally about enhancing patient safety and well-being. By utilizing AI for tasks such as identifying potential failures or successes, accelerating patient selection for clinical studies, and unraveling discoveries in disease pathology, the entire drug development pipeline becomes more effective. This effectiveness translates into better, more targeted treatments for patients.
Furthermore, AI’s capacity to handle vast amounts of data and rapidly scale up processes presents a significant opportunity in drug discovery. Collaboration, an essential part of scientific discovery, is bolstered by AI, promoting interdisciplinary collaboration and enhancing decision-making processes.
Future-readiness and Adaptability
Embracing generative AI in drug discovery also requires organizations to be future-ready and adaptable. This involves not just adopting new technologies but also reengineering workflows and ecosystems to accommodate the power of AI. The potential of AI to augment innovation and creativity is immense, freeing scientists from mundane tasks and allowing them to focus on the creative and innovative aspects of their work, which could lead to groundbreaking discoveries in the field.
Generative AI is rapidly becoming a mainstay in the biopharma industry strategy, with its adoption expected to double in the next 3-5 years. This trend signifies a new era in drug discovery, particularly in addressing “hard-to-drug” indications and targets, promising more drug candidates entering the clinic in the near future.
In conclusion, generative AI in drug discovery is reshaping the way we approach medical research, enhancing efficiency, reducing costs, and most importantly, improving patient outcomes. Its role is pivotal in the current and future landscape of pharmaceutical research and development.
FAQ Section:
- What is Generative AI in drug discovery?
- Generative AI in drug discovery refers to the use of advanced AI techniques to generate new drug molecules and predict their properties.
- How does Generative AI improve the drug discovery process?
- It accelerates molecular design, enhances target identification, predicts drug interactions, aids in drug repurposing, and facilitates personalized medicine.
- What technologies underpin Generative AI?
- Technologies like deep learning, machine learning, and neural networks form the core of Generative AI in drug discovery.
- Can Generative AI reduce the time taken for drug development?
- Yes, by rapidly analyzing vast data sets and predicting outcomes, Generative AI can significantly reduce the drug development timeline.
- Is Generative AI effective in identifying drug targets?
- Yes, it excels in analyzing complex biological data to identify and validate new targets for drug development.
- How does Generative AI impact personalized medicine?
- It enables the customization of drug therapies based on individual genetic profiles, enhancing treatment effectiveness.
- What are the challenges faced in implementing Generative AI?
- Challenges include ensuring data accuracy, overcoming computational limitations, and addressing ethical concerns.
- Does Generative AI have a role in drug repurposing?
- Absolutely, it can identify new therapeutic uses for existing drugs, thereby saving time and resources.
- How does Generative AI ensure patient safety?
- By accurately predicting drug interactions and side effects, it plays a crucial role in enhancing patient safety.
- What is the future of Generative AI in drug discovery?
- It’s poised for significant growth, with advancements in AI technology continuing to transform drug development processes.