Last Updated on April 23, 2024 12:34 pm by Laszlo Szabo / NowadAIs | Published on April 23, 2024 by Laszlo Szabo / NowadAIs
See the Unseen: Adobe’s VideoGigaGAN AI Model Brings Stunning Detail to Blurry Videos – Key Notes
- Adobe’s VideoGigaGAN AI model significantly enhances the resolution of low-resolution videos, ensuring fine details and temporal consistency.
- Builds on the technology of GigaGAN for image upsampling, extended to videos.
- Uses an asymmetric U-Net architecture with additional temporal attention layers to maintain consistency across frames.
- Overcomes common VSR issues like flickering artifacts and aliasing with advanced modules like flow-guided propagation.
- Demonstrates superior performance in upscaling videos up to 8× resolution while preserving intricate details and realistic textures.
Introduction
Video Super-Resolution (VSR) has long been a challenging task in the fields of computer vision and graphics. The goal is to enhance the resolution of low-resolution videos, recovering fine details and ensuring temporal consistency across frames. While previous VSR approaches have shown impressive temporal consistency, they often fall short in generating high-frequency details, leading to blurry results compared to their image counterparts.
Adobe, a leading innovator in the field, has introduced VideoGigaGAN, a generative AI model that addresses this fundamental challenge. Building upon the success of their large-scale image upsampler, GigaGAN, VideoGigaGAN takes video super-resolution to new heights, delivering videos with astonishing detail and smooth consistency.
The Challenge of Video Super-Resolution
Achieving both temporal consistency and high-frequency details in video super-resolution poses a significant challenge. Previous approaches have focused on maintaining temporal consistency by using regression-based networks and optical flow alignment techniques. While these methods ensure smooth transitions between frames, they often sacrifice the generation of detailed appearances and realistic textures. This limitation has motivated researchers at Adobe to explore the potential of Generative Adversarial Networks (GANs) in video super-resolution.
VideoGigaGAN: Extending the Success of Image Upsampling to Videos
VideoGigaGAN builds upon the strong foundation of GigaGAN, a large-scale GAN-based image upsampler developed by Adobe. GigaGAN leverages billions of images to model the distribution of high-resolution content and generate fine-grained details in upsampled images. By inflating GigaGAN into a video model and incorporating temporal modules, VideoGigaGAN aims to extend the success of image upsampling to the challenging task of video super-resolution while preserving temporal consistency.
Method Overview: Enhancing Detail and Consistency
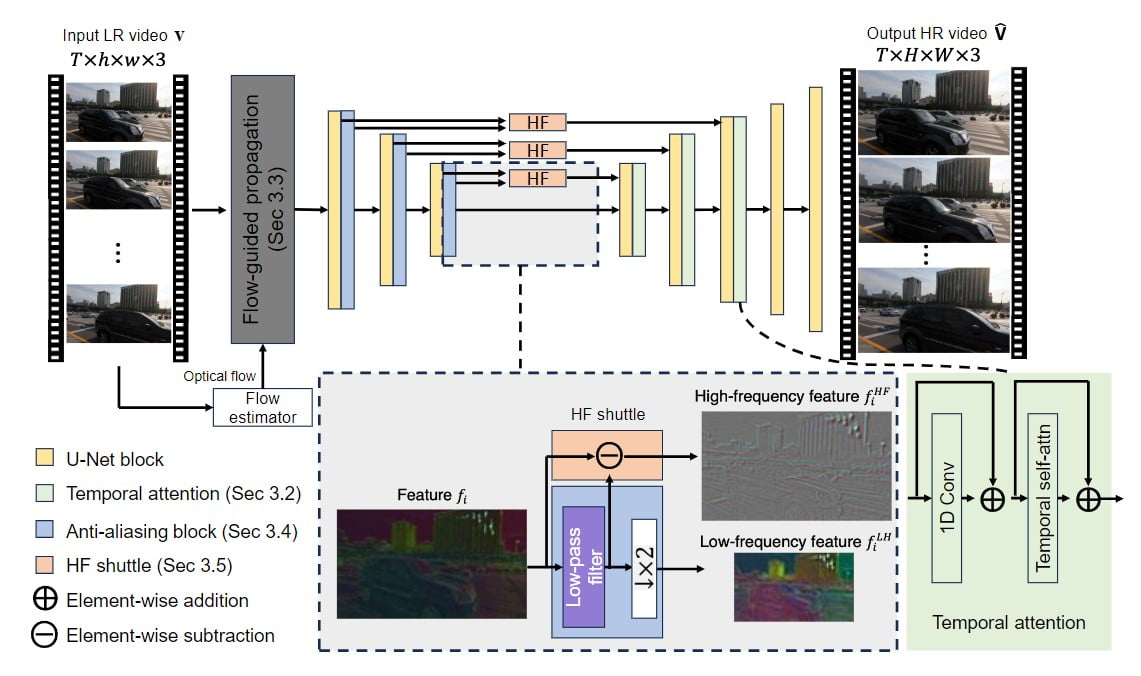
The architecture of VideoGigaGAN is based on the asymmetric U-Net, similar to the image GigaGAN upsampler. However, to enforce temporal consistency, several key techniques have been introduced. First, the image upsampler is inflated into a video upsampler by adding temporal attention layers into the decoder blocks. This ensures that the generated frames are consistent over time.
Additionally, a flow-guided propagation module is incorporated to enhance consistency across frames by aligning features based on flow information. To suppress aliasing artifacts caused by downsampling, anti-aliasing blocks are introduced in the encoder. Moreover, high-frequency features are directly shuttled to the decoder layers via skip connections to compensate for detail loss during the upsampling process.
Ablation Study: Overcoming Flickering Artifacts and Aliasing
In the ablation study, the researchers at Adobe identified the strong hallucination capability of image GigaGAN as a cause of temporally flickering artifacts, especially aliasing resulting from the low-resolution input. To address these issues, several components were progressively added to the base model. These components include temporal attention layers, flow-guided feature propagation, anti-aliasing blocks, and the high-frequency shuttle. Through these additions, VideoGigaGAN achieves a remarkable balance between detail-rich upsampling and temporal consistency, mitigating the artifacts and flickering observed in previous approaches.
Comparison with State-of-the-Art Methods
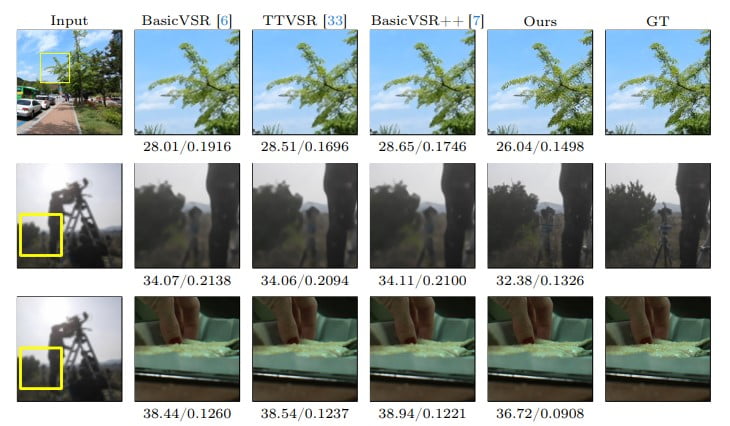
VideoGigaGAN has been extensively evaluated and compared to state-of-the-art video super-resolution models on public datasets. The results demonstrate that VideoGigaGAN generates videos with significantly more fine-grained appearance details while maintaining comparable temporal consistency. By leveraging the power of GANs and incorporating innovative techniques, VideoGigaGAN pushes the boundaries of video super-resolution, offering a new level of realism and quality in upscaled videos.
Upsampling Results: Unveiling Unparalleled Detail
One of the most impressive aspects of VideoGigaGAN is its ability to upscale videos by up to 8× while preserving stunning detail. The model excels in generating high-frequency details that were not present in the low-resolution input, resulting in videos with a remarkable level of realism and clarity. By comparing the upsampling results with ground truth images, it is evident that VideoGigaGAN outperforms previous methods in terms of detail preservation and overall quality.
A Glimpse into the Future: Handling Generic Videos and Small Objects
VideoGigaGAN’s capabilities extend beyond specific video categories. The model demonstrates impressive performance in handling generic videos with diverse content. However, challenges remain when it comes to extremely long videos and small objects. Future research can focus on improving optical flow estimation and addressing the challenges associated with handling small details like text and characters. By further refining these aspects, VideoGigaGAN has the potential to unlock even greater possibilities in video super-resolution.
Conclusion: Changing Video Upscaling with VideoGigaGAN
Adobe’s VideoGigaGAN AI model represents a significant advancement in the field of video super-resolution. By leveraging the power of GANs and incorporating innovative techniques, VideoGigaGAN achieves unparalleled detail and consistency in upscaled videos. With its ability to upscale videos by up to 8× while maintaining fine details and temporal stability, VideoGigaGAN opens up new possibilities for enhancing low-resolution video content. As the demand for high-quality video continues to grow across various industries, VideoGigaGAN has the potential to revolutionize how we process and consume video media.
Definitions
- Video Super-Resolution (VSR): The process of converting low-resolution video to higher resolution by recovering fine details and ensuring smooth frame-to-frame transitions.
- GigaGan: A powerful generative adversarial network developed by Adobe for upscaling images, known for its ability to enhance image details significantly.
- VideoGigaGAN: An extension of GigaGan adapted for video, which uses advanced AI techniques to improve the resolution and quality of videos while maintaining temporal consistency.
- Temporal consistency in videos: The smoothness and uniformity of sequential frames in a video, ensuring that enhancements do not disrupt the flow of motion or introduce jarring visual artifacts.
- Asymmetric U-Net: A neural network architecture used for tasks like image segmentation, adapted in VideoGigaGAN for handling both spatial and temporal dimensions of video data.
- State-of-the-Art Methods: The most advanced and effective techniques currently available in a field, often setting benchmarks for performance and efficiency.
Frequently Asked Questions
- What sets Adobe’s VideoGigaGAN apart from other video super-resolution tools? Adobe’s VideoGigaGAN employs a unique combination of GAN technology and a novel U-Net architecture, enabling it to enhance video resolution while maintaining a high level of detail and temporal consistency unmatched by previous methods.
- Can Adobe’s VideoGigaGAN handle videos with fast motion or complex scenes? Yes, VideoGigaGAN is designed to handle a variety of video content, including fast motion and complex scenes, thanks to its flow-guided modules and temporal attention layers that ensure smooth and detailed outputs.
- What are the technical requirements to use Adobe’s VideoGigaGAN AI model effectively? Using Adobe’s VideoGigaGAN AI model requires a robust computing setup, ideally with high-performance GPUs like the NVIDIA RTX series, to handle the intensive processing needed for real-time video enhancement.
- How does Adobe’s VideoGigaGAN ensure the realism and quality of upscaled videos? VideoGigaGAN incorporates advanced AI techniques such as generative adversarial networks, temporal attention, and flow-guided propagation to enhance videos realistically, ensuring that the upscaled videos retain natural textures and coherent motion.
- What future improvements can be expected with Adobe’s VideoGigaGAN AI model? Future versions of VideoGigaGAN will likely focus on improving its efficiency, reducing computational demands, and enhancing its ability to handle even longer videos and smaller objects with greater precision.