Last Updated on August 12, 2024 12:54 pm by Laszlo Szabo / NowadAIs | Published on August 12, 2024 by Laszlo Szabo / NowadAIs
Goodbye, Turn-Based AI: Hello Listening-While-Speaking Language Model – Key Notes
- The Listening-While-Speaking Language Model (LSLM) integrates listening and speaking in real time, eliminating the constraints of turn-based dialogue systems.
- Developed by Shanghai Jiao Tong University and ByteDance, LSLM uses a dual-channel architecture combining token-based TTS and streaming SSL encoder.
- LSLM handles interruptions and background noise efficiently, demonstrating robustness and sensitivity in various experimental settings.
- Middle fusion strategy optimizes interaction by merging listening and speaking channels at each Transformer block, ensuring a seamless dialogue experience.
Introduction
In the landscape of human-computer interaction (HCI), the quest for more natural and intuitive communication has been a driving force behind technological advancements. As the most fundamental form of human interaction, dialogue has long been the holy grail for conversational AI systems. Recent breakthroughs in speech language models (SLMs) have undoubtedly enhanced the capabilities of speech-based conversational AI, yet these systems have remained constrained by their turn-based nature, lacking the ability to engage in real-time, uninterrupted interactions.
This limitation has sparked a renewed focus on exploring full duplex modeling (FDM) in interactive speech language models (iSLM), with researchers seeking to unlock the quintessential ability of interruption and seamless back-and-forth communication. Amidst this pursuit, a fresh innovation has emerged: the Listening-While-Speaking Language Model (LSLM), an end-to-end system designed to update the way humans and machines converse.
The Limitations of Turn-Based Dialogue Systems
Traditional speech-language models have typically relied on a turn-based approach, where listening and speaking occur in isolated phases. This siloed structure, often involving separate automatic speech recognition (ASR) and text-to-speech (TTS) modules, has led to inherent latency issues and an inability to handle real-time interruptions effectively. Notable models like SpeechGPT and LauraGPT have pushed the boundaries of conversational AI, but they remain limited to these turn-based paradigms, failing to provide the fluid interaction required for truly natural human-computer dialogue.
The Birth of LSLM: Bridging the Gap in Real-Time Interaction
Recognizing the need for a more seamless and responsive conversational experience, a team of researchers from Shanghai Jiao Tong University and ByteDance introduced the Listening-While-Speaking Language Model (LSLM). This model aims to overcome the limitations of turn-based systems by integrating both listening and speaking capabilities within a single, end-to-end architecture.
The Dual-Channel Approach of LSLM
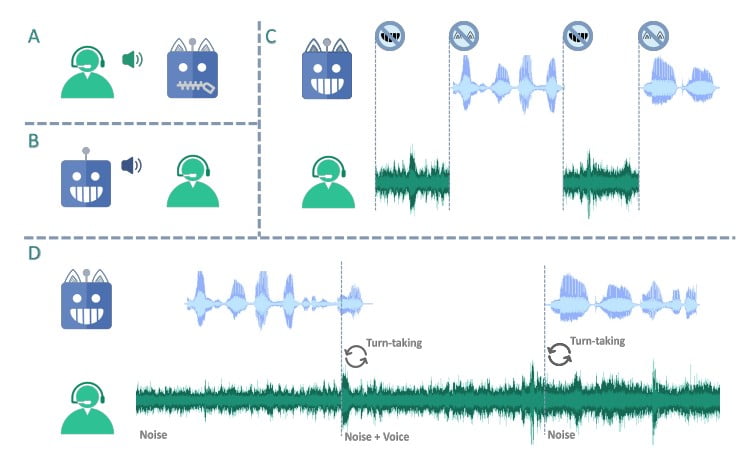
The LSLM’s unique design revolves around its dual-channel architecture, combining a token-based decoder-only TTS for speech generation and a streaming self-supervised learning (SSL) encoder for real-time audio input. This approach allows the model to fuse the listening and speaking channels, enabling it to detect turn-taking in real-time and respond dynamically to the user’s input.
The Speaking Channel: Autoregressive Token-Based TTS
Departing from previous models that relied on autoregressive and non-autoregressive approaches, the LSLM simplifies the speech generation process by utilizing a token-based TTS system. This setup allows the model to focus more on semantic information, improving the clarity and relevance of its responses while enhancing real-time interaction by eliminating the need for extensive pre-processing before speech synthesis.
The Listening Channel: Streaming SSL Encoder
On the listening side, the LSLM employs a streaming SSL encoder to process incoming audio signals continuously. This encoder converts the audio input into continuous embeddings, which are then projected into a space that can be seamlessly integrated with the speaking tokens. This integration ensures that the model can leverage both channels’ information throughout the speech generation process.
Fusion Strategies: Balancing Real-Time Interaction and Speech Generation
To optimize the synergy between the listening and speaking channels, the researchers explored three fusion strategies: early fusion, middle fusion, and late fusion. After careful evaluation, the middle fusion approach emerged as the most effective, striking an optimal balance between real-time interaction and speech generation capabilities.
In the middle fusion method, the listening and speaking channels are merged at each Transformer block, allowing the model to continuously leverage the insights from both channels during the speech generation process. This integration ensures that the LSLM can handle interruptions smoothly and maintain a coherent and responsive dialogue flow, adapting to the user’s input in real-time.
Evaluating LSLM’s Performance: Robustness and Sensitivity
The LSLM’s capabilities were put to the test in two experimental settings: command-based FDM and voice-based FDM. In the command-based scenario, the model demonstrated its robustness to background noise, effectively responding to specific commands amidst a noisy environment. The voice-based setting, on the other hand, evaluated the LSLM’s sensitivity to interruptions from various speakers, showcasing its ability to recognize and adapt to new voices and instructions.
The results of these experiments highlighted the LSLM’s impressive performance, underscoring its potential to revolutionize the field of interactive speech-language models. The middle fusion strategy, in particular, proved to be a crucial factor in balancing the demands of real-time interaction and speech generation, delivering a seamless and responsive user experience.
Advancing the Frontiers of Conversational AI
The Listening-While-Speaking Language Model (LSLM) represents a significant leap forward in the realm of interactive speech-language models. By addressing the limitations of turn-based systems and introducing a robust, real-time interaction capability, the LSLM paves the way for more natural and fluid human-computer dialogues. This research highlights the importance of integrating full duplex capabilities into SLMs, showcasing how such advancements can enhance the applicability of conversational AI in real-world scenarios.
Conclusion: Unlocking the Full Potential of Conversational AI
The Listening-While-Speaking Language Model (LSLM) represents a transformative breakthrough in the field of interactive speech-language models. By seamlessly integrating listening and speaking capabilities, this design overcomes the limitations of traditional turn-based systems, ushering in a new era of more natural and fluid human-computer dialogue. As the demand for intuitive and responsive conversational AI continues to grow, the LSLM’s ability to facilitate real-time interaction and handle interruptions with ease positions it as a changer in the pursuit of truly seamless human-AI communication.
Descriptions
- Full Duplex Modeling (FDM): A communication system where both parties can speak and listen simultaneously, unlike turn-based models where one party must wait for the other to finish speaking.
- Token-Based Decoder-Only TTS: A system that uses tokens, or bits of data, to generate speech, allowing the AI to respond more quickly and accurately by focusing on meaning rather than pre-processing extensive data.
- Streaming Self-Supervised Learning (SSL) Encoder: A type of AI that processes audio inputs continuously, converting sounds into data that can be understood and used by the model for real-time interaction.
- Transformer Block: A component in AI models that helps process and understand language by focusing on different parts of the input data simultaneously, improving speed and accuracy.
- Fusion Strategies: Techniques used to integrate data from different channels in an AI model. Early, middle, and late fusion strategies determine how and when data is combined during processing to optimize performance.
- Command-Based FDM: An experimental setup where the AI model responds to specific voice commands, testing its ability to operate amidst background noise and interruptions.
- Voice-Based FDM: An experimental scenario evaluating how well the AI can handle different voices and interruptions, assessing its adaptability to new speakers and instructions.
Frequently Asked Questions
- What is the Listening-While-Speaking Language Model (LSLM)? The Listening-While-Speaking Language Model (LSLM) is an advanced AI system designed to engage in real-time dialogue by integrating listening and speaking capabilities. Unlike traditional models, it allows for seamless back-and-forth communication without the need for turn-taking.
- How does the LSLM handle interruptions during conversations? LSLM uses a dual-channel architecture with a streaming SSL encoder that continuously processes audio input. This setup allows it to recognize and adapt to interruptions smoothly, maintaining a coherent dialogue flow even when new voices or commands are introduced.
- What makes the middle fusion strategy effective in the LSLM? The middle fusion strategy merges listening and speaking channels at each Transformer block, allowing the model to leverage both sets of information throughout the dialogue. This approach balances real-time interaction with speech generation, enhancing the AI’s responsiveness and coherence.
- How does the LSLM manage background noise during its operations? In command-based experimental settings, the LSLM demonstrated its robustness by effectively filtering out background noise and focusing on specific commands. Its advanced processing capabilities ensure accurate responses even in noisy environments.
- What are the potential applications of the Listening-While-Speaking Language Model? The LSLM can enhance human-computer interactions in various fields, including customer service, smart home devices, and virtual assistants. Its ability to handle real-time dialogue and interruptions makes it ideal for scenarios requiring seamless and intuitive communication.